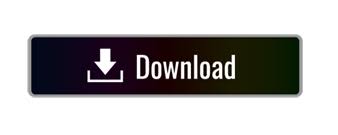
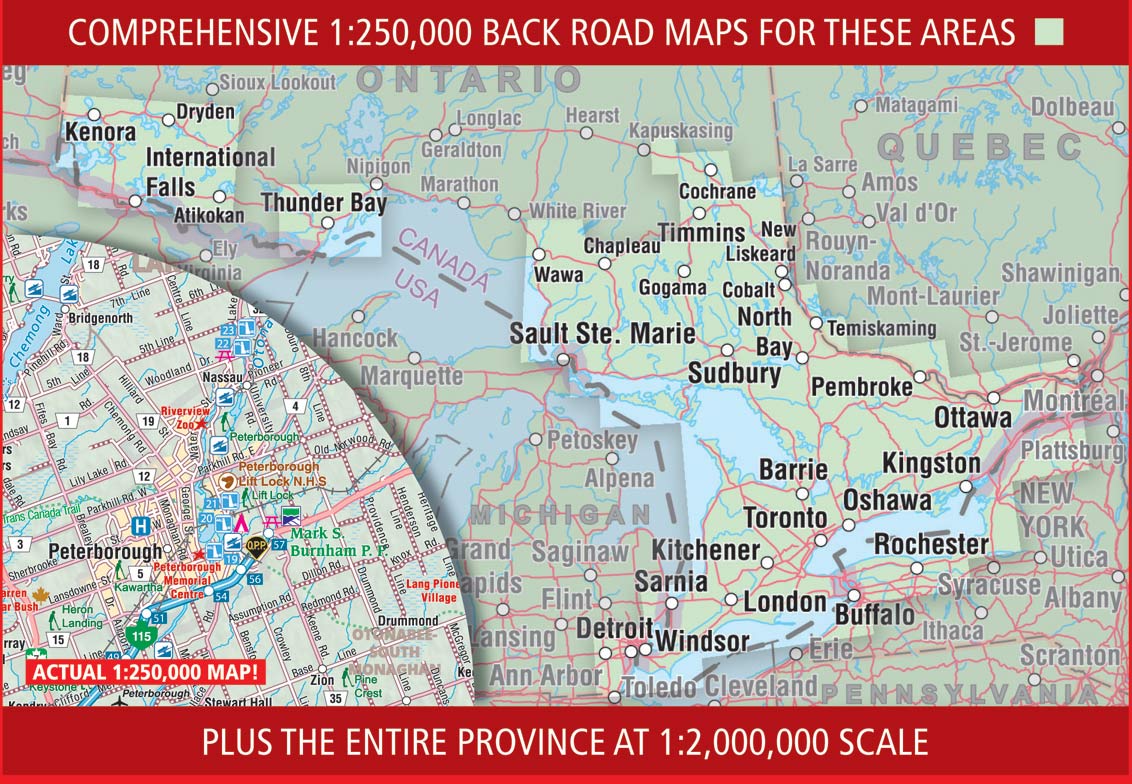
Unfortunately, Bing has a few disadvantages. You just have to click on a traffic light icon on a city systems to access that traffic and camera data. Bing Maps, does show traffic information. While Bing Maps uses HERE, it doesn't offer all of HERE's winning features. HERE, on the other hand, provides street data from various third-party sources. Bing Maps offers bird's eye views, directions and satellite images. Originally Nokia's Navtez's, "HERE", a word play of being "here" partly powers Bing Maps today.
#Street atlas 2015 optimize route software#
Bing MapsĮven Bing Maps was conceptualized in 2005, it was then known as a simple mapping software Microsoft MapPoint, and TerraServer which was a compilation of U.S.
#Street atlas 2015 optimize route android#
It's predominantly found on Android where it's, in fact, a default application on various devices. While Google does overlay traffic data, it does not provide information on traffic cameras. In addition to this, Google also works with transit providers to capture real-time transit data. Their Ground Truth team constantly improves existing Maps using the details acquired from local images like street signs, numbers on houses and turn lane signs.

Google also collects ground data from their street view cameras. Census Bureau's TIGER for data, it now gets data from various sources including MAPIT and Tele Atlas. If you wonder which is better of the two, here's a rundown on the two maps, and their features, to help you make your selection. However, there's also an alternative for those who don't like being stuck with just one option. Google Maps is the first choice most people make, which isn't surprising because it's dominating today. With so many places to search, and so many routes to reach them, mapping things save time and minimizes inconvenience. What food is nearby? What entertainment is close to here? Retailers have been using this process for years in order to optimize their sight selection. More people are staring to use the search feature on maps to see what is around them. Maps - Companies need them not only to guide customers to their outlet but for various other reasons too - like searching what is available near them. Modeling bicycling from Strava and geographic data enables mapping of bicycling ridership that is more inclusive of all bicyclists and better able to support decision-making.2020 February 18 By Real Clear Software Google Maps vs. Counts collected continuously over a long time period, rather than at peak periods, may also improve modeling. For best results, official count data should capture diverse street conditions, including low ridership areas. However, model accuracy was influenced most by the official count data used for model training.


Inclusion of city-specific variables increased model performance, as the R 2 for generalized and city-specific models ranged from 0.08–0.80 and 0.68–0.92, respectively. Our results indicate generalized prediction of bicycling ridership on a road segment in concert with Strava data should include the following variables: number of Strava riders, percentage of Strava trips categorized as commuting, bicycling safety, and income. Using the LASSO-selected variables as predictors in Poisson regression, we built generalized and city-specific models and compared accuracy. Using a machine learning approach, LASSO, we identify variables important for predicting ridership in all cities, and independently in each city. We used Strava data, official counts, and geographic data to model Average Annual Daily Bicycling (AADB) in five cities: Boulder, Ottawa, Phoenix, San Francisco, and Victoria. In doing so we enable detailed mapping that is more inclusive of all bicyclists and will support more equitable decision-making across cities. Our goal is to develop a generalized approach to modeling bicycling ridership using Strava data. However, studies conducted on single cities provide limited insight on best practices for modeling bicycling with Strava as generalizability is difficult to assess. To overcome bias introduced by data generated from only fitness app users, researchers build statistical models that predict total bicycling by integrating Strava data with official counts and geographic data. Fitness apps, such as Strava, are a growing source of data for mapping bicycling ridership, due to large samples and high resolution.
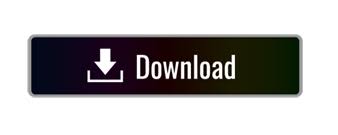